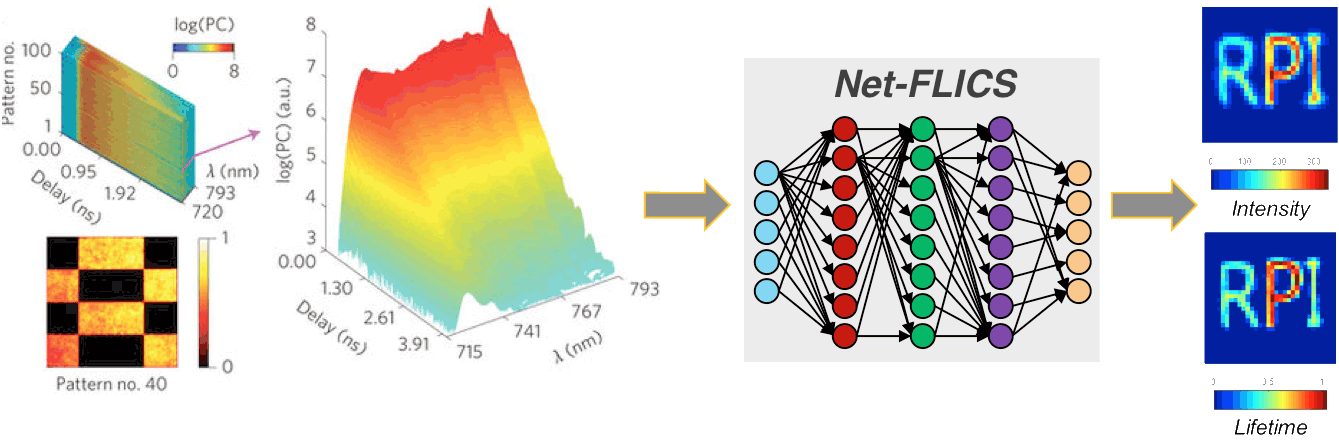
Compressive Macroscopic Fluorescence Lifetime Imaging (MFLI) is a novel technical implementation that allows multiple molecular interactions in macroscopic scale to be modeled. A hyperspectral wide-field time-resolved single-pixel imaging platform was recently developed that facilitates whole-body in vivo lifetime imaging in less than 14 minutes. However, despite efficient data acquisition, the data processing of a Compressed Sensing (CS) based inversion plus lifetime fitting remain very time consuming. Herein, we investigate the potential of deep learning for fast and accurate image formation. More precisely, we developed a Convolutional Neural Network (CNN) called Net-FLICS (Network for Fluorescence Lifetime Imaging with Compressive Sensing) that reconstructs both intensity and lifetime images directly from raw CS measurements. Results show that better quality reconstruction can be obtained using Net-FLICS, for both the simulation and experimental datasets. This is completed in a fraction of the time needed for a traditional analytic method. This first investigation suggests that Net-FLICS may be a powerful tool that may enable CS-based lifetime imaging for real-time applications. This is a collaborative project that was developed jointly with Prof. Xavier Intes’ group at RPI.